Thesis by Daniel Carlos Da Silva: « Développement d'une méthodologie de modélisation analytique des composants électriques dans un véhicule hybride : Optimisation des performances énergétiques » (Development of a methodology for the analytical modeling of the electric components in a hybrid vehicle: energy performance optimization).
To maximize the contribution of hybrid electric vehicles (HEV) to the decarbonization of the transport sector, their energy performance is a key factor that needs to be considered from the design phase. For an HEV, optimizing the design in order to reduce consumption involves not only optimizing a sizing aspect but also the EMS1. This co-optimization of sizing and energy management is generally tackled either by nesting optimal control algorithms within an optimization algorithm dedicated to sizing, or by using convex optimization2 to simultaneously optimize the design levels. However, the former approach is known to induce computational constraints (for example relating to calculation time), while the latter can affect modeling fidelity due to the constraints inherent in convex optimization.
Adopting a new perspective, this PhD research introduced a methodology for estimating powertrain energy consumption using a fully explicit formulation, illustrated in Figure 1. This was in the form of an EPCM3, initially developed using component models, to consider power losses, and then predictive models, located at a second modeling level, in order to take into account the impact of component sizing variations.
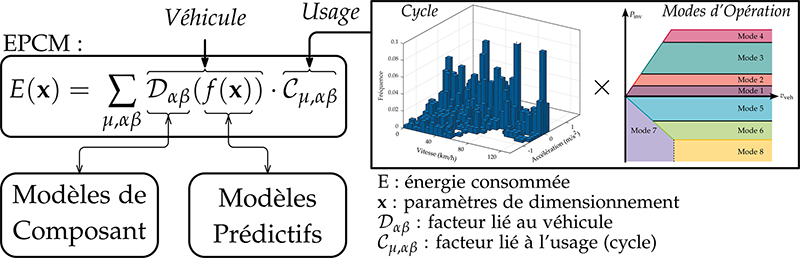
The new methodology was developed using an FCHEV4 as the reference. For vehicle energy analyses relating to the system as a whole, the research focused not only on modeling the components commonly found in electric vehicles, but also on modeling the power electronics and fuel cell system [1], aspects often neglected or inadequately addressed.
The component models were developed and validated using data obtained from a Toyota Mirai II during tests on IFPEN’s roller test benches. Analysis of hydrogen consumption over test cycles demonstrated a global error between measurement and model of less than 5 percent [2]. Operating data sets for components of various sizes were then used to develop predictive models based on regression analyses. Compared with the traditional use of performance maps, which are highly specific to each component, more general models like this introduced an error of less than three percent on hydrogen consumption. [3].
By exploring the use of the EPCM developed for the FCHEV, instead of an optimal control law, this thesis demonstrated that the use of an EMS employing a simple affine function provides a reasonable approximation for co-optimization in the early stages of vehicle design.
A computational performance analysis then showed that using the EPCM in a sizing optimization problem can reduce evaluation time by a factor of 200, while retaining both the sizing obtained with conventional modeling and an optimal EMS (Figure 2.a). This new formulation was also studied on reduced sizing problems5, allowing us to obtain linear and quadratic expressions to approximate the hydrogen consumption obtained with a conventional formulation (Figure 2.b).
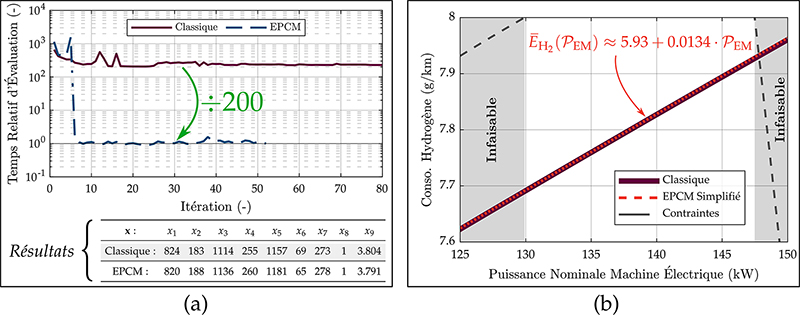
(a) Comparison of evaluation time and optimization results using a conventional formulation and the EPCM.
(b) Comparison of the estimation of hydrogen consumption obtained using a conventional formulation and a simplified EPCM.
Once developed, such simple models can be used to draw rapid conclusions about the energy performance of an electric vehicle, and can be coupled with other key aspects of vehicle design, such as cost and life-cycle analysis.
1- Energy Management Strategy.
2- Where the objective function is convex (https://fr.wikipedia.org/wiki/fonction_convexe), as well as the feasible set (https://fr.wikipedia.org/wiki/Ensemble_convexe), which significantly facilitates optimization.
3- Explicit Powertrain Consumption Model.
4- Fuel Cell Hybrid Electric Vehicle.
5- i.e., with a reduced number of sizing variables, e.g., one or two.
6- i.e., using a quasi-static model for each component, with an optimal control law.
References:
-
D. Carlos da Silva, L. Kefsi and A. Sciarretta, An analytical model to optimize the powertrain sizing of Fuel Cell Hybrid Electric Vehicles, 2022 IEEE Vehicle Power and Propulsion Conference (VPPC), Merced, CA, USA.
>> https://doi.org/10.1109/VPPC55846.2022.10003436
-
D. Carlos da Silva, L. Kefsi and A. Sciarretta, Analytical Models for the Sizing Optimization of Fuel Cell Hybrid Electric Vehicle Powertrains, 16th International Conference on Engines & Vehicles for Sustainable Transport (ICE2023), Capri – Naples, Italy.
>> https://doi.org/10.4271/2023-24-0133
-
D. Carlos da Silva, L. Kefsi and A. Sciarretta, Closed-Form Expression to Estimate the Hydrogen Consumption of a Fuel Cell Hybrid Electric Vehicle, IEEE Transactions on Vehicular Technology, 2024.
>> https://doi.org/10.1109/TVT.2024.3350351
Scientific contact: daniel.carlos-da-silva@ifpen.fr